筛选
科学数据
统计数据
共检索到23条 ,权限内显示50条;
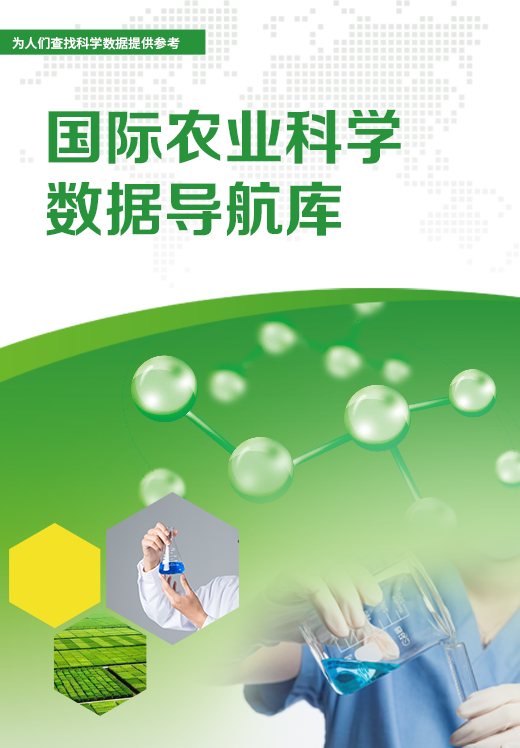
Data from: Morbidity rate prediction of dengue hemorrhagic fever (DHF) using the support vector machine and the Aedes aegypti infection rate in sim
- 负责人:
- 关键词:
- DOI:
- doi:10.5061/dryad.078bn
- 摘要:
- ng the support vector machine (SVM) technique for forecasting the dengue morbidity rate. Methods and Findings: We identified the study areas in three provinces (Nakhon Pathom
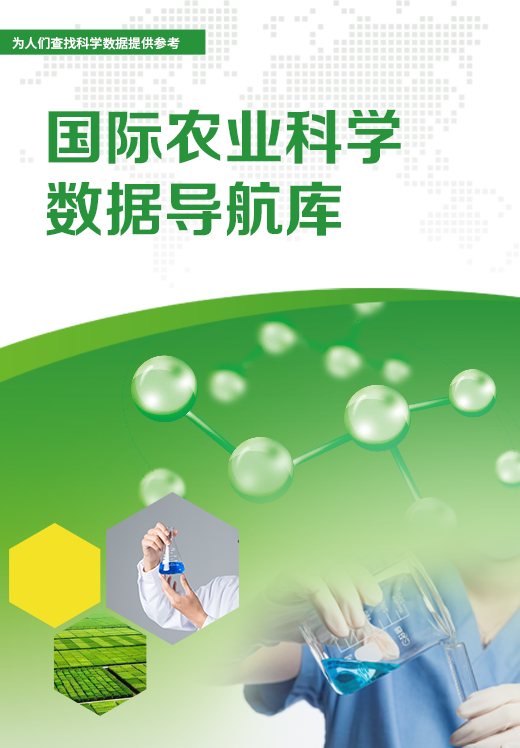
Data from: Pain intensity recognition rates via biopotential feature patterns with support vector machines
- 负责人:
- DOI:
- doi:10.5061/dryad.2b09s
- 摘要:
- : The machine learning measurement of pain in patients could provide valuable information for a clinical team and thus support the treatment assessment.
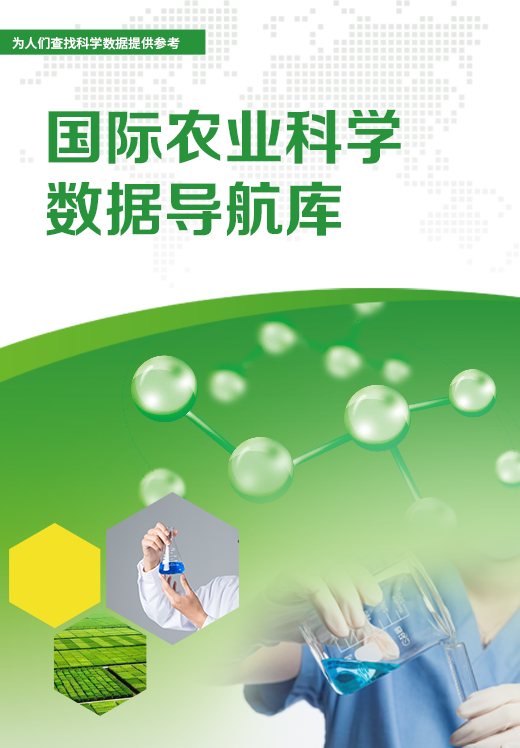
Data from: Vis/NIR reflectance spectroscopy for hybrid rice variety identification and chlorophyll content evaluation for different
- 负责人:
- DOI:
- doi:10.5061/dryad.p8pq7fq
- 摘要:
- ls. The support vector machine (SVM) algorithm was applied to identify five varieties of hybrid rice and six levels of nitrogen fertilizer. The results demonstrated tha
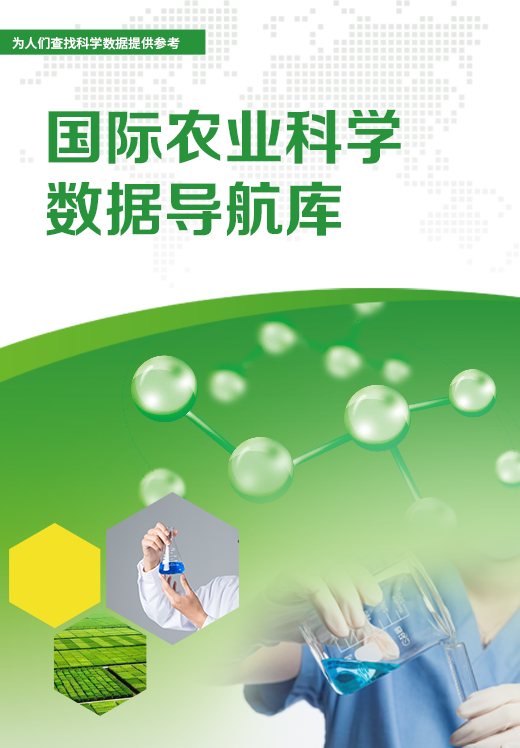
Data from: Development of machine learning models for diagnosis of glaucoma
- 负责人:
- DOI:
- doi:10.5061/dryad.q6ft5
- 摘要:
- ion model, we considered four machine learning algorithms: C5.0, random forest (RF), support vector machine (SVM), and k-nearest neighbor (KNN). We repeatedly compo
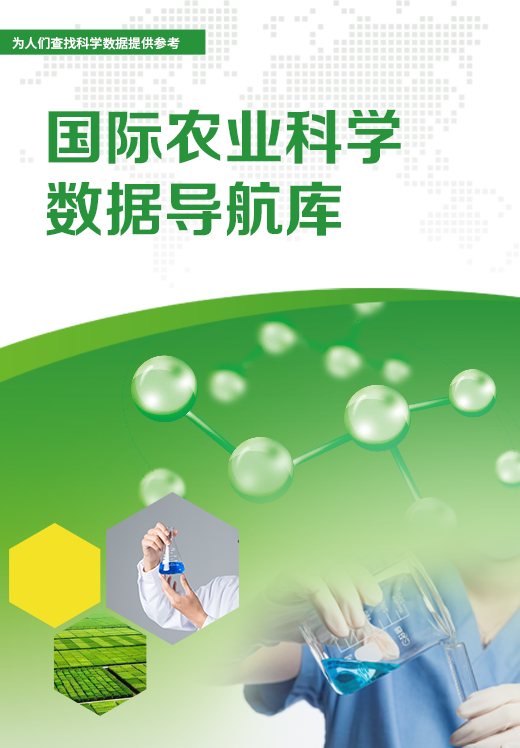
Data from: Evaluating internal model strength and performance of myoelectric prosthesis control strategies
- 负责人:
- DOI:
- doi:10.5061/dryad.v12f25n
- 摘要:
- . The performance of both strategies was also evaluated using a Schmidt’s style target acquisition task. Results obtained from 24 able-bodied subjects showed that alt
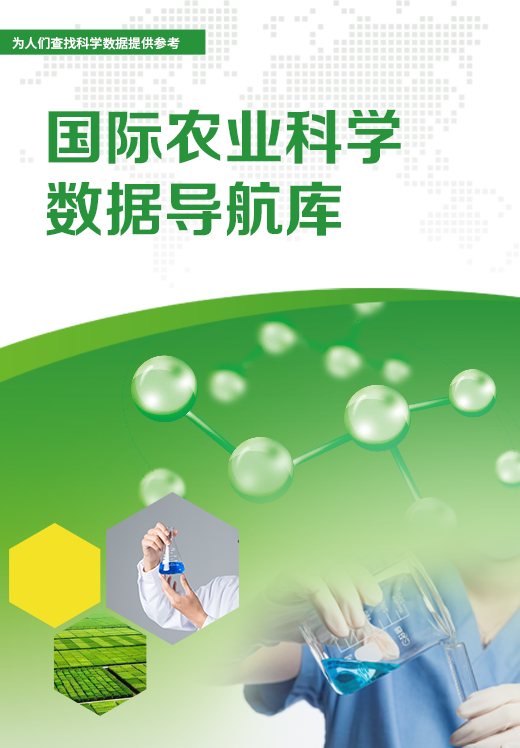
Patchy promiscuity: machine learning applied to predict the host specificity of Salmonella enterica and Escherichia coli
- 负责人:
- University Of Edinburgh;;University Of Edinburgh
- DOI:
- doi:10.7488/ds/2102
- 摘要:
- Support Vector Machine (SVM) classifiers were built based on whole genome sequence content. Analysis of over 1000 S. enterica genomes allowed the correct prediction (67% - 90
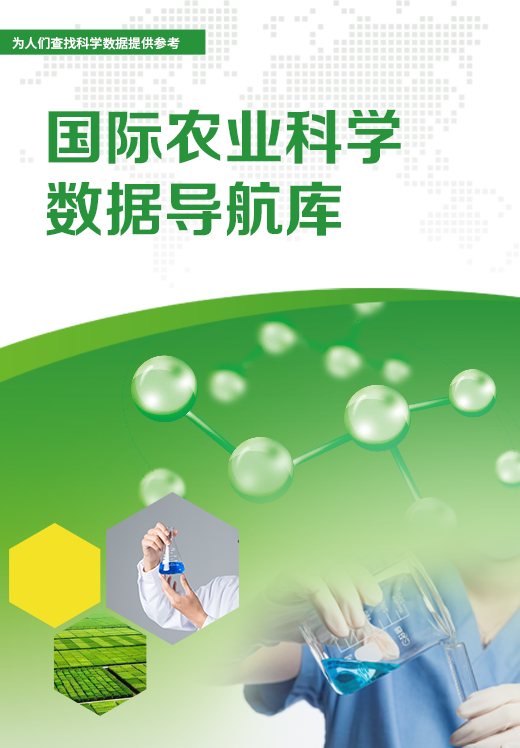
Non-animal methods to predict skin sensitization (II): an assessment of defined approaches**<\/sup>
- 负责人:
- 关键词:
- Genetics Physiology Biotechnology 59999 Environmental Sciences not elsewhere classified Immunology 69999 Biological Sciences not elsewhere classified 80699 Information Systems not elsewhere classified Science Policy
- DOI:
- doi:10.6084/m9.figshare.5933323.v2
- 摘要:
- , for both hazard and potency characterization. Defined approaches examined included consensus methods, artificial neural networks, support vector machine
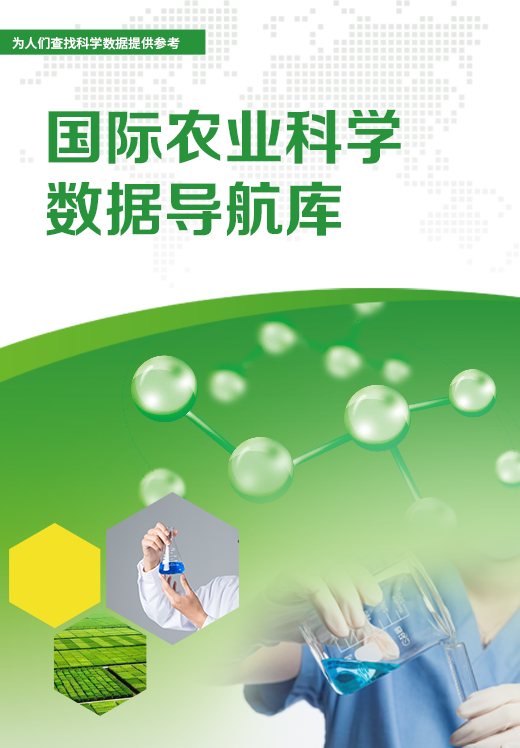
Data from: A novel algorithm to enhance P300 in single trials: application to lie detection using F-score and SVM
- 负责人:
- DOI:
- doi:10.5061/dryad.2qc64
- 摘要:
- nce comparison. The optimal parameter values in the SDA and the classifiers were tuned using a grid-searching training procedure with cross-validation. The support vector machine
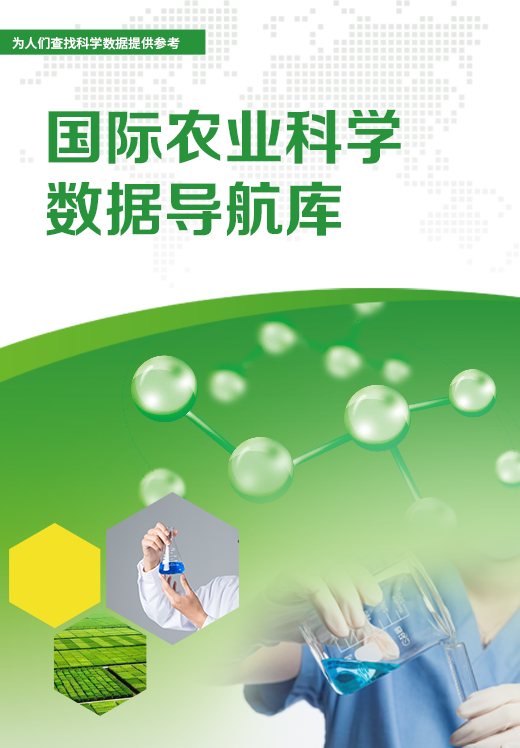
Data from: In-vivo imaging of cell migration using contrast enhanced MRI and SVM based post-processing
- 负责人:
- 关键词:
- DOI:
- doi:10.5061/dryad.vh5ht
- 摘要:
- cell localization method using contrast enhanced multiparametric MRI and support vector machines (SVM) based post-processing. Imaging phantoms consisting of agarose
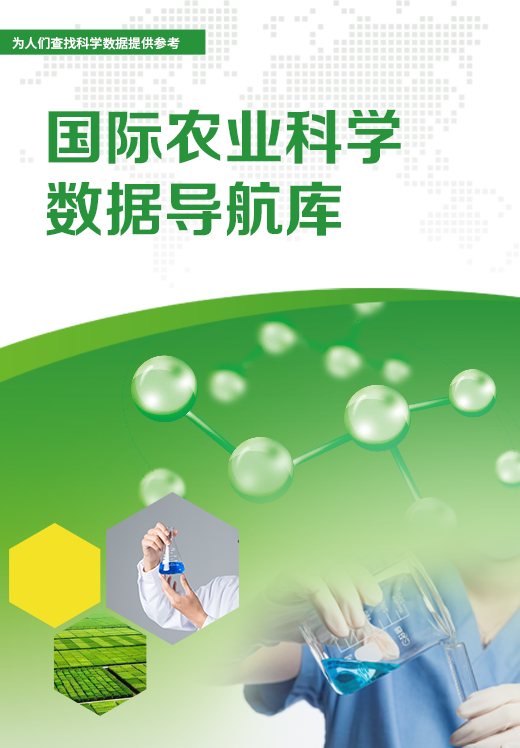
Data from: Predicting classifier performance with limited training data: applications to computer-aided diagnosis in breast and prostate cancer
- 负责人:
- DOI:
- doi:10.5061/dryad.m5n98
- 摘要:
- , the relationships between 3 distinct classifiers (k-nearest neighbor, naive Bayes, Support Vector Machine) are explored. Further quantitative evaluation in terms